Why AI agents are dominating enterprise tech conversations
"With AI agent systems, it’s not about being ‘all-knowing’ - it’s about ‘exactly knowing.’"
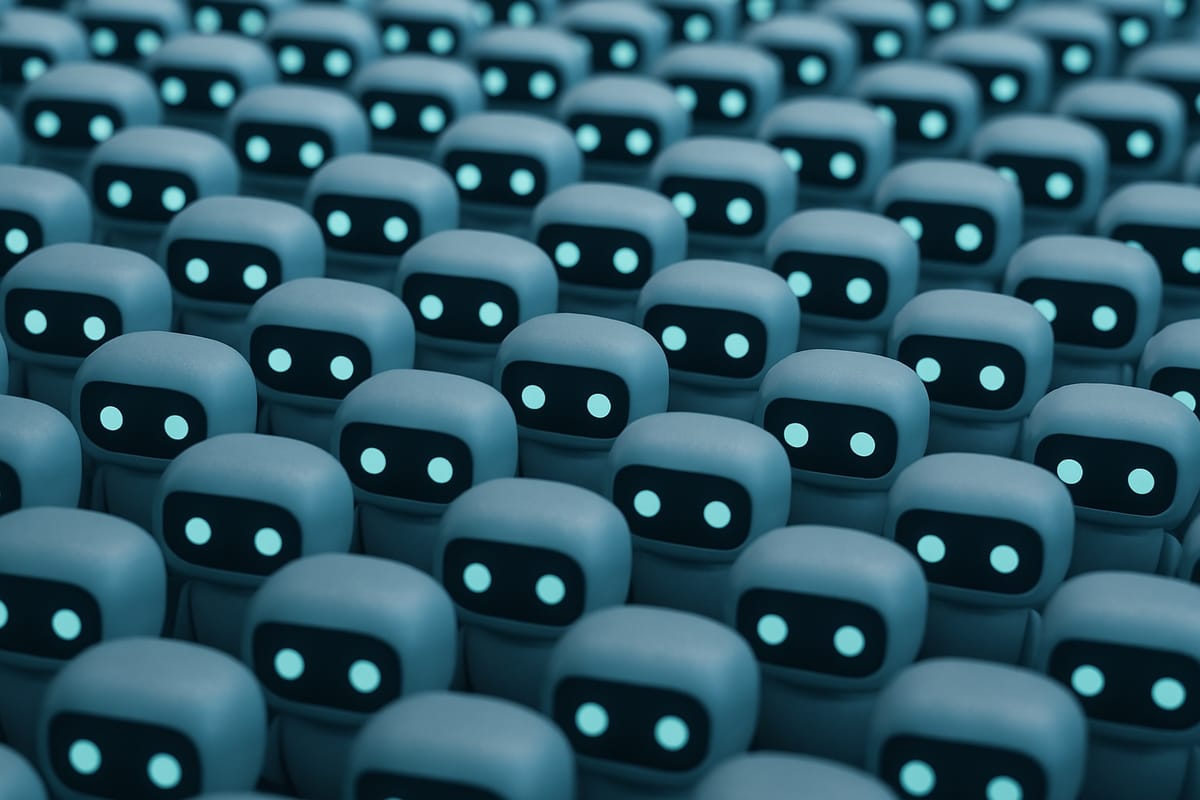
AI agents have emerged as the latest buzzword in tech circles - dominating headlines and conference discussions as businesses seek more practical applications of generative AI.
The surge in attention reflects a growing challenge businesses face transitioning Gen AI projects from pilot to full-scale production due to privacy, quality, and cost concerns. It also stems from a growing need for them to have more than just ‘general knowledge’ of their data - instead delivering contextually relevant, highly accurate, and trustworthy insights tailored to their unique data landscape and business requirements.
But what’s so special about them and how can businesses extract the most value?
From ‘general intelligence’ to data intelligence
With AI agent systems, it’s not about being ‘all-knowing’; it’s about ‘exactly knowing’.
General-purpose models like ChatGPT are extremely useful in many contexts, but when deployed on their own, they can struggle to deliver meaningful results for enterprises. Trained on broad datasets, these models are often too generic to fully grasp the unique language, processes, and data structures that define a specific business.
The real opportunity lies in using the right model for the right job. That might be an open-source model fine-tuned for a particular domain, or a commercial model integrated into a broader framework of tools and components. What matters most is not the model itself, but how well it is applied to solve a clearly defined business problem.
AI agents enable this by coordinating specialised components – from retrieval and reasoning to validation and orchestration – each tailored to a specific function. For example, a customer support agent and a financial forecasting agent might operate within the same system, but each is optimised for its own domain and supported by relevant, private data.
This targeted approach allows businesses to move beyond the limitations of general intelligence, and towards data intelligence; the ability to reason on their own proprietary data and drive informed decisions.
Building trust through validation and transparency
Trust remains a significant barrier to AI adoption, with many UK businesses hesitant to deploy systems prone to errors, biases, or unpredictable outputs. AI agent systems tackle this challenge head-on by incorporating both human oversight and AI-based validation mechanisms.
Many businesses implementing agent systems employ "human-in-the-loop" approaches combined with sophisticated evaluation tools that cross-check and refine outputs before deployment.
These layers of validation create more trust. For enterprises, this means smoother adoption, greater confidence, and better outcomes.
The data foundation imperative
The effectiveness of AI agent systems hinges on robust data infrastructure. As businesses race to transform into data-driven enterprises, they face significant challenges; stakeholder pressure to adopt AI without clear starting points, fragmented datasets across the organisation, and heightened governance and security concerns.
Despite these obstacles, forward-thinking companies are making progress through carefully scoped pilot projects that demonstrate return on investment before scaling. This measured approach helps build the necessary people, processes, and technology foundation for sustainable AI transformation.
A key part of successful AI transformation is also about bringing data intelligence to the forefront. Businesses can do this through modern data architectures - such as data intelligence platforms - which unify, govern, and operationalise data in one place. With natural language interfaces and private data integration, businesses can build custom models that truly understand their specific needs. These systems empower non-technical employees to interact with data more easily, democratising AI and accelerating adoption across teams.
An Economist Impact report underscores this trend, with nearly 60% of surveyed professionals expecting natural language to become the primary or sole method for non-technical employees to interact with complex datasets within three years.
The future belongs to AI agent systems and a new era of precision
The future of enterprise AI is about crafting systems of specialised AI agents working in harmony. This shift from general to specific intelligence enables businesses to address unique challenges with greater precision and confidence.
Businesses can build their own AI agent systems tailored to their specific needs when supported by the right data and AI platform. With this, companies can harness their proprietary data to create customised, domain-specific AI agent systems that deliver reliable, high-quality outputs. By combining tools like vector databases for precise data retrieval, fine-tuning or prompting for domain-specialised reasoning, and monitoring frameworks to ensure safety and compliance, businesses can craft intelligent applications uniquely suited to their business goals.
As the conversation around AI continues to evolve, AI agent systems represent not just a technological advancement but a fundamental shift in how businesses conceive of and implement artificial intelligence. For businesses ready to move beyond general AI models, these systems offer a path to more focused, trustworthy, and valuable AI-driven outcomes.
Courtney Bennett is Director of Field Engineering at Databricks