Microsoft admits new Phi-4 small language model is "tedious"
Waffly model delights in giving "long and elaborate" answers that can be just a little bit dull.
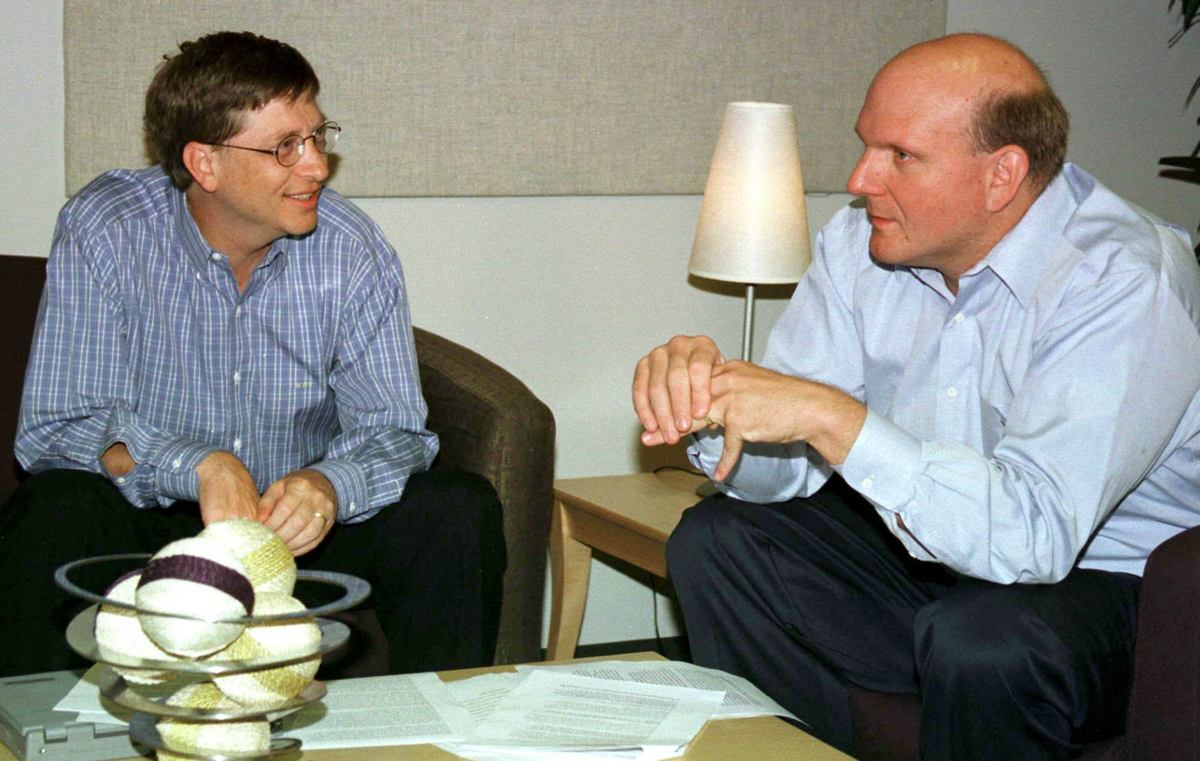
Microsoft has confessed that interactions with its latest lean language model Phi-4 can be somewhat dull.
Today, the totally-not-boring tech giant announced the launch of a small language model (SLM) trained on a relatively modest 14 billion parameters that "offers high-quality results at a small size."
Ece Kamar, VP and Lab Director of AI Frontiers, said the new SLM "continues to push the frontier of size vs quality".
"Phi-4 outperforms comparable and larger models on math-related reasoning due to advancements throughout the processes, including the use of high-quality synthetic datasets, curation of high-quality organic data, and post-training innovations," she wrote.
You can see its mathematical reasoning in the image below.
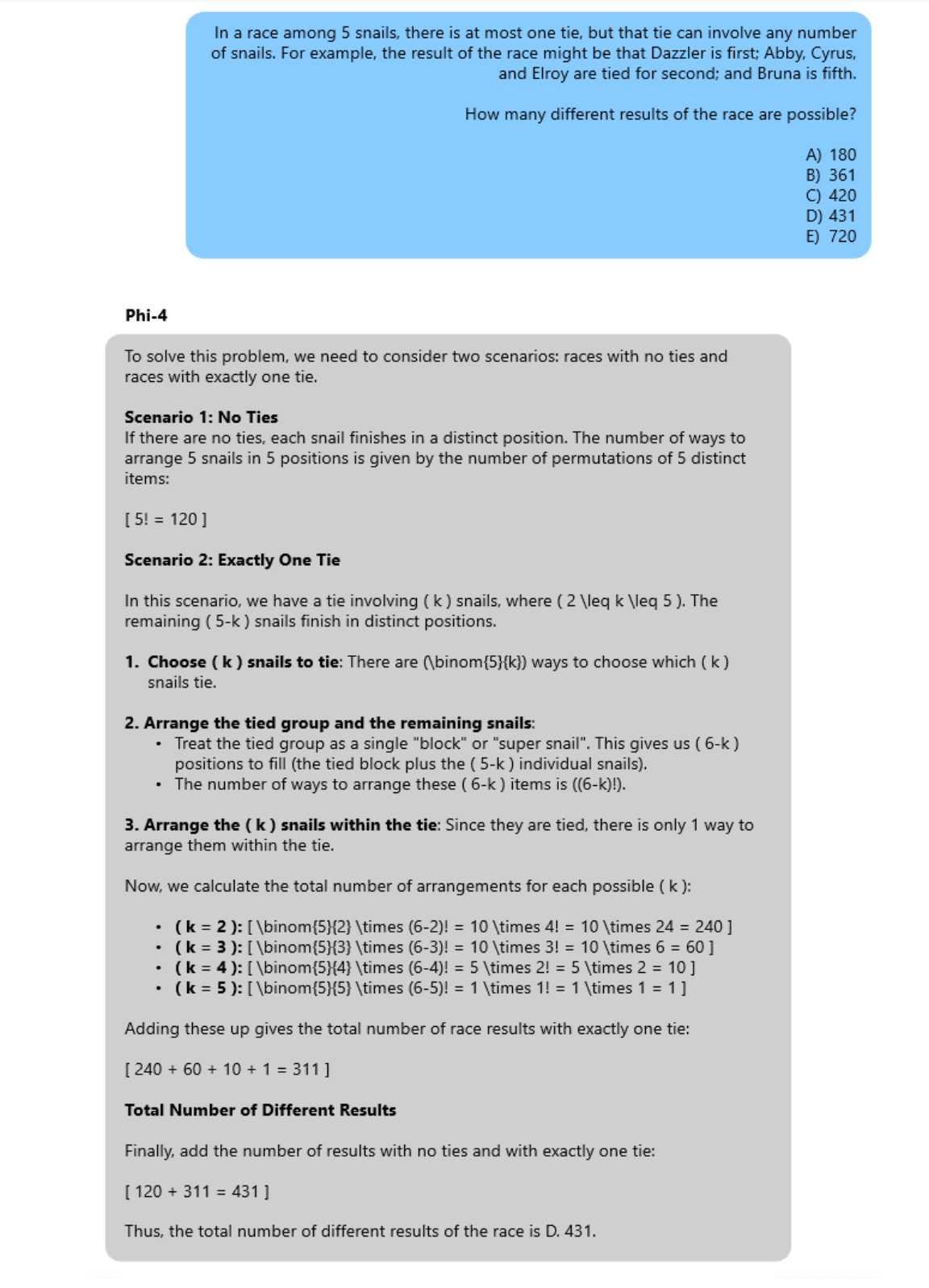
Phi, fi, no fun
Although Phi-4 is great for maths classes and accountancy sessions, you probably wouldn't like to be stuck next to it at a party.
"As our data contains a lot of chain-of-thought examples, phi-4 sometimes gives long elaborate answers even for simple problems - this might make user interactions tedious," Microsoft wrote in its technical notes.
Chain of thought simulates human reasoning by breaking tasks into a series of small steps which must be completed to achieve a final answer.
Phi-4 is also "still fundamentally limited by its size for certain tasks" - specifically in "hallucinations around factual knowledge", Microsoft also revealed.
"For example, if X is a plausible human name, the model sometimes responds to prompts of the form 'Who is X?' with a hallucinated biography of the person X," the Office titan added. "This limitation would be improved by augmenting the model with a search engine, but factual hallucinations cannot be eliminated completely."
However, in this case, you've gotta blame the game and not the player. Small models will never be as effective as their large ones - but point to an exciting future of on-device AI action in which lean models perform relatively simple tasks without needing to be connected to the Internet.
A spokesperson for Jenova.ai contacted us on Blue Sky to share the following assessment of Phi-3's capabilities: "We've been testing Phi-4 extensively while integrating it into jenova ai's model router. While it's efficient and accurate, it lacks creative flair. That's why we dynamically route creative tasks to more expressive models like Claude 3.5 or GPT-4o. Different tools for different jobs!"
Phi-4: A small but mighty language model
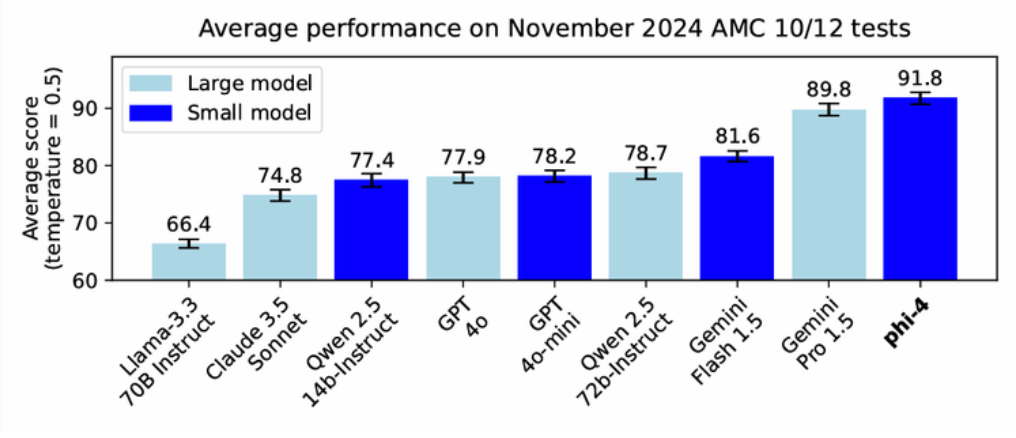
The model performs impressively, although it can get flummoxed by questions like "which number is smaller, 9.9 or 9.11?" - picking the larger number.
"While phi-4 demonstrates relatively strong performance in answering questions and performing reasoning tasks, it is less proficient at rigorously following detailed instructions, particularly those involving specific formatting requirements," Microsoft confessed.
This means it had difficulties making tables, bullet points or "precisely matching stylistic constraints", often producing outputs that deviate from the specified guidelines."
So Phi-4 is not only a bit of a windbag. It's also far from guaranteed to always do what it's told.
However, despite these grumbles, the new Microsoft model appears to be a high achiever - which is down to the training material it grew up on.
Training of the 14-billion parameter language model focused on feeding it with data of the best possible quality.
"Unlike most language models, where pre-training is based primarily on organic data sources such as web content or code, phi-4 strategically incorporates synthetic data throughout the training process," Microsoft wrote.
"While previous models in the Phi family largely distil the capabilities of a teacher model (specifically GPT-4), phi-4 substantially surpasses its teacher model on STEM-focused QA capabilities, giving evidence that our data-generation and post-training techniques go beyond distillation."
How is Phi-4 different from Phi-3?
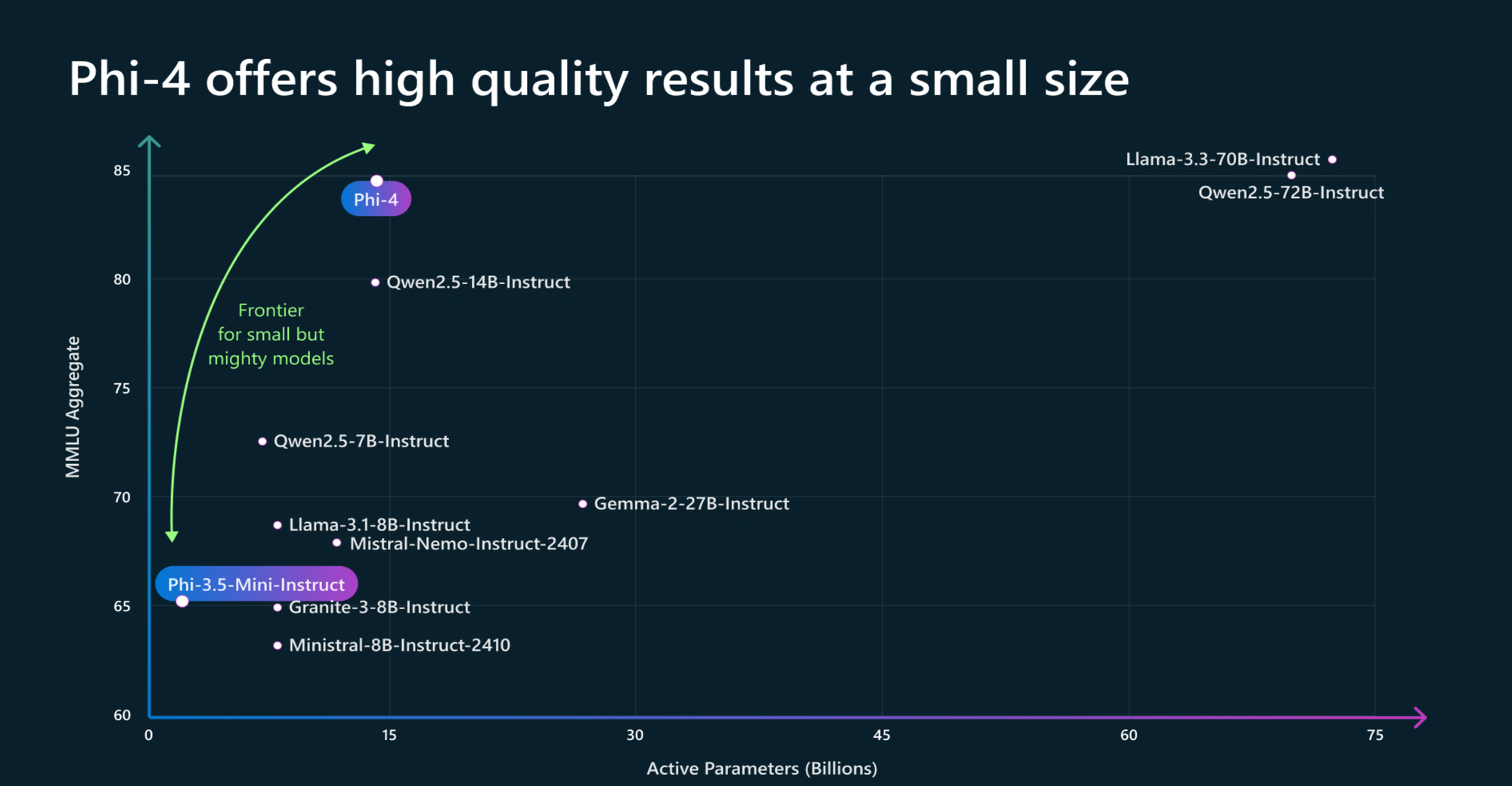
Only "minimal changes" were made to the phi-3 architecture, resulting in a remarkable performance relative to its size – especially on reasoning-focused benchmarks. Microsoft said this was directly down to "improved data, training curriculum, and innovations in the post-training scheme."
Synthetic data makes up the bulk of the training data for phi-4 and is generated with a range of techniques including multi-agent prompting (using multiple AI agents to collaborate or compete on tasks), self-revision workflows (AI improving its own outputs iteratively) and instruction reversal (rephrasing or reversing the instructions to explore alternate solutions or understand a problem better).
"These methods enable the construction of datasets that induce stronger reasoning and problem-solving abilities in the model, addressing some of the weaknesses in traditional unsupervised datasets," Microsoft wrote.
Techniques such as rejection sampling (filtering outputs based on specific criteria) and Direct Preference Optimization (DPO - training models to align directly with user preferences) help to "refine the model’s outputs".
The life of Phi
Phi-4 is guided by three core pillars:
1. Synthetic data for pretraining and midtraining: High-quality datasets prioritise reasoning and problem-solving. They are "carefully generated to ensure diversity and relevance, incorporating changes to the training curriculum and new pretraining and midtraining data mixtures to "increase the allocation of synthetic tokens".
2. Curation and filtering of high-quality organic data: Microsoft "meticulously" curated and filtered data sources, including web content, licensed books, and code repositories to extract seeds for the synthetic data pipeline that "encourage high-depth reasoning and prioritize educational value (to the model)".
3. Post-training: The post-training recipe in Phi-4 used new refined supervised fine-tuning versions of SFT datasets, as well as developing a new technique to create DPO pairs, based on pivotal token search.
"With these innovations, the performance of Phi-4 on reasoning-related tasks is comparable to or surpasses much larger models," Microsoft wrote.
Its performance on reasoning-related benchmarks "meets or exceeds" Llama-3.1-405B and "significantly exceeds its teacher GPT-4o on the GPQA (graduate-level STEM Q&A) and MATH (math competition) benchmarks.
The phi-4 model demonstrates substantial improvements in safety and grounding while maintaining low levels of harmful outputs.
But we still can't forget that Microsoft admission that it's a little tedious. A great performer, sure, but a bit of a bore. What else could be described in the same way, we wonder?
Have you got a story to share? Get in touch and let us know.