Open-AI co-founder Ilya Sutskever: Peak Data is here and the end of pre-training is nigh
AI pioneer and safe superintelligence visionary argues that the era of using ever-bigger datasets to train ever-larger neural networks is over.
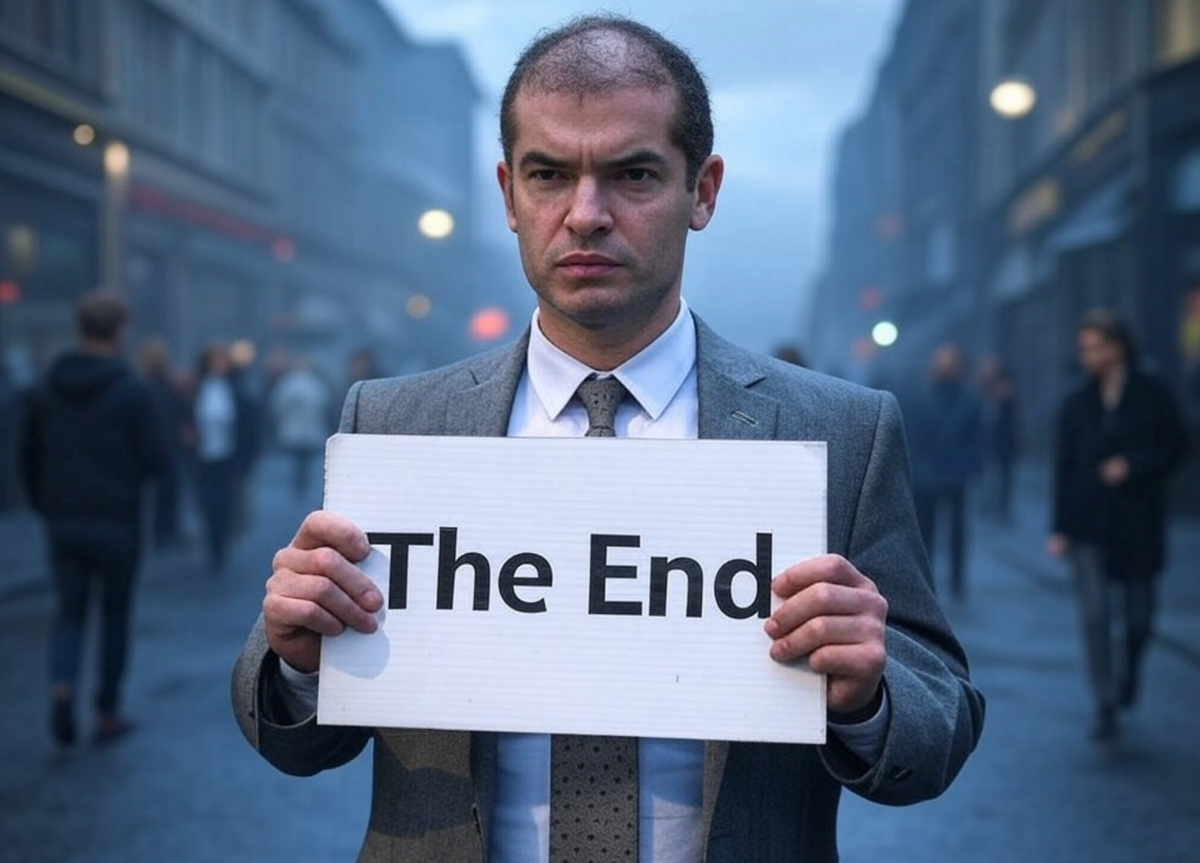
The man who helped to found OpenAI before launching his own bid to develop safe superintelligence has announced that we are at the end of an AI era.
Ilya Sutskever, co-founder and former chief scientist at Open AI, said that the industry is reaching "Peak Data" as human civilisation literally runs out of the main resource used to power the AI revolution so far: data from the internet.
In a talk at NeurIPS 2024, a conference on neural information processing systems, Sutskever compared this powerful but highly polluted dataset to crude oil. We know that burning it is bad, but we don't have an alternative and need to keep the lights on.
Sutskever's fascinating presentation is a sequel to a speech he gave more than 10 years ago. We added some then-and-now shots underneath to remind you of the passage of time (sorry).
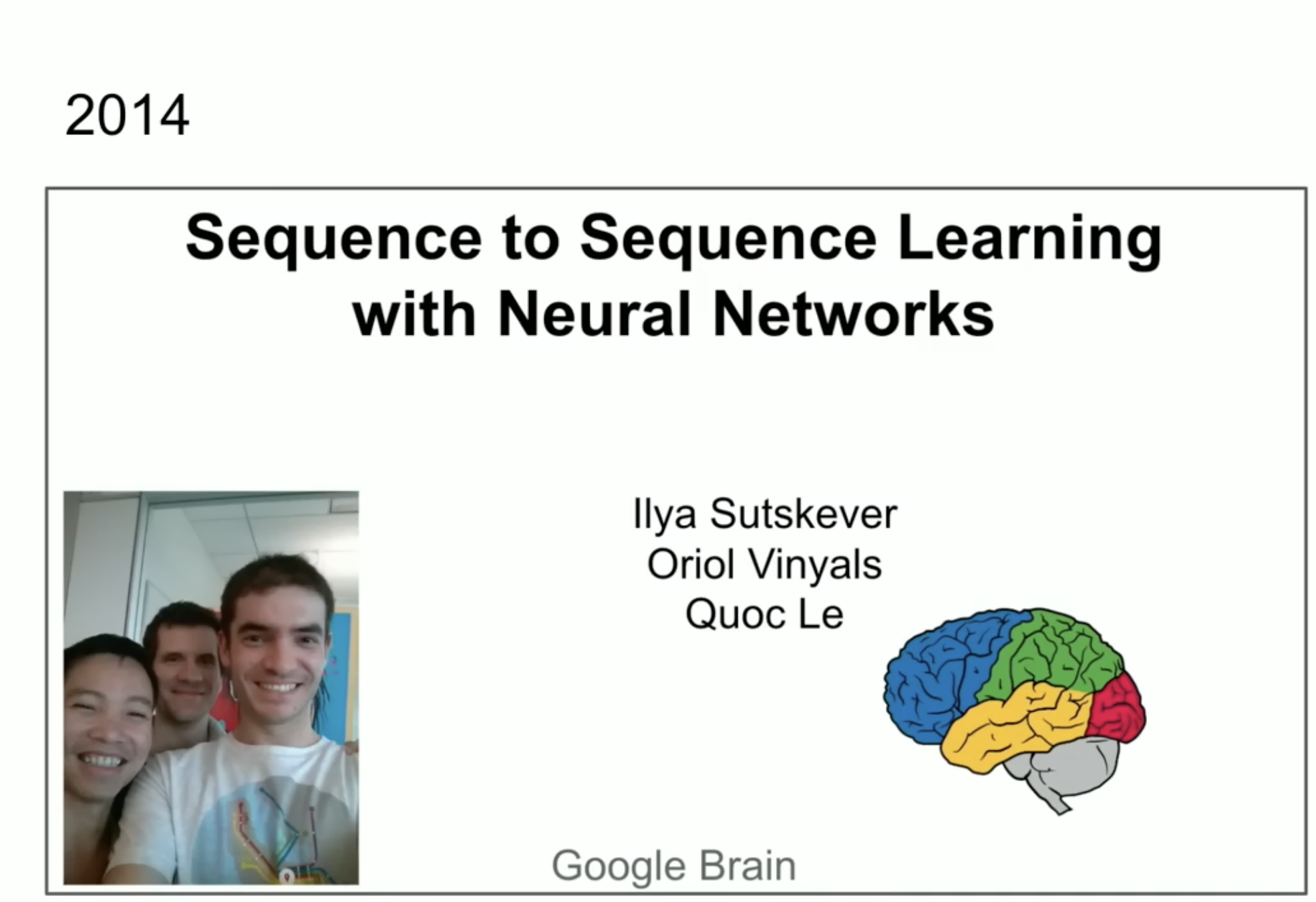
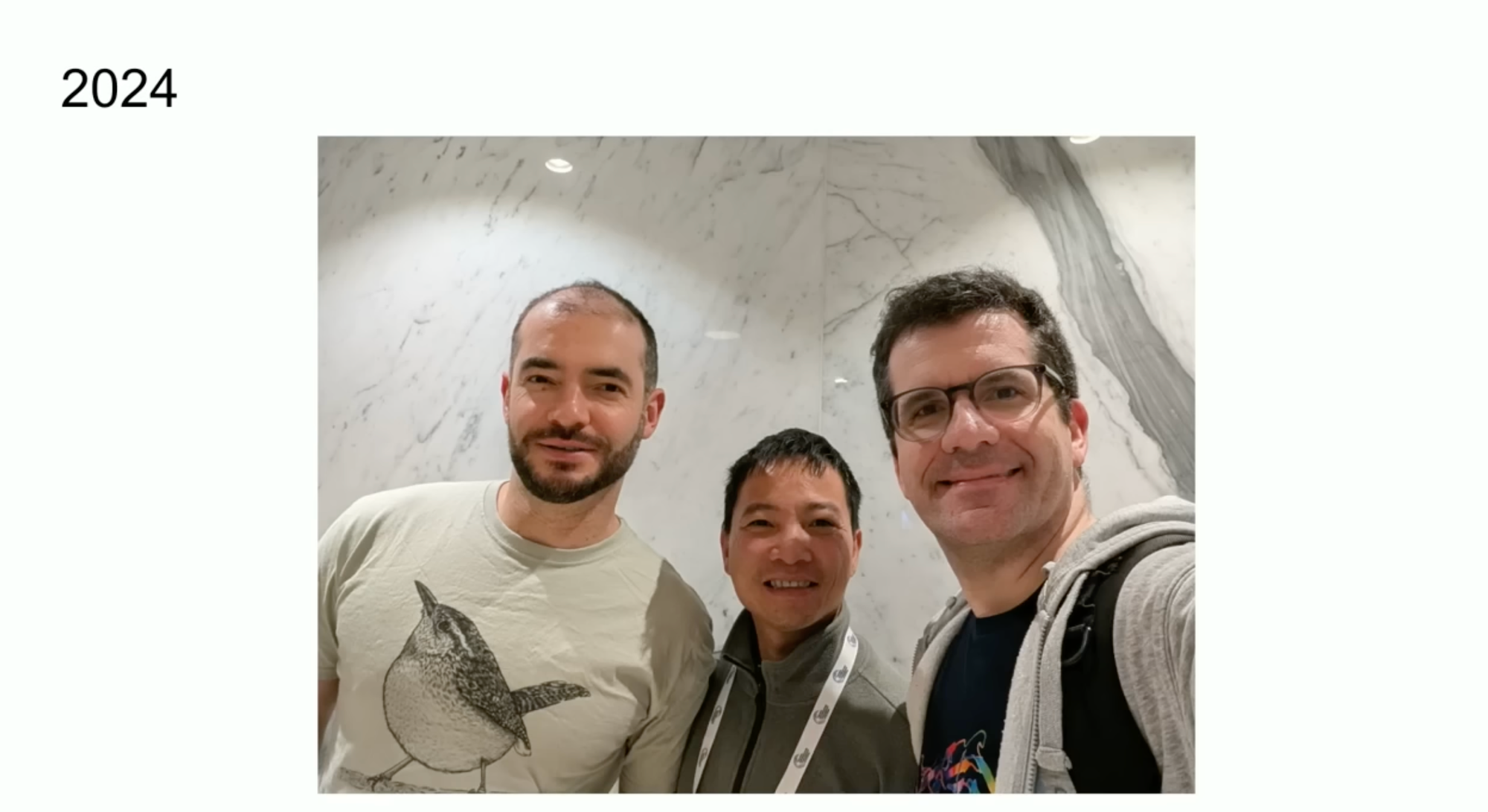
In that presentation a decade ago, the distinguished computer scientist discussed three innovations that we'll explain later in the article:
- Big data sets
- Large neural networks
- Autoregressive models trained on text
Sutskever said one of the things he got right back then was the "deep learning hypothesis".
"This model suggested that a large neural network with 10 layers could "do anything a human can do," he remembered.
Another argument that has "stood the test of time" is connectionism, which is the idea that artificial neurons are similar enough to biological neurons that large neural networks can replicate many human capabilities.
However, unlike human brains, AI lacks the ability to self-reconfigure and relies heavily on large datasets, making humans superior in adaptability.
What is an autoregressive AI model?
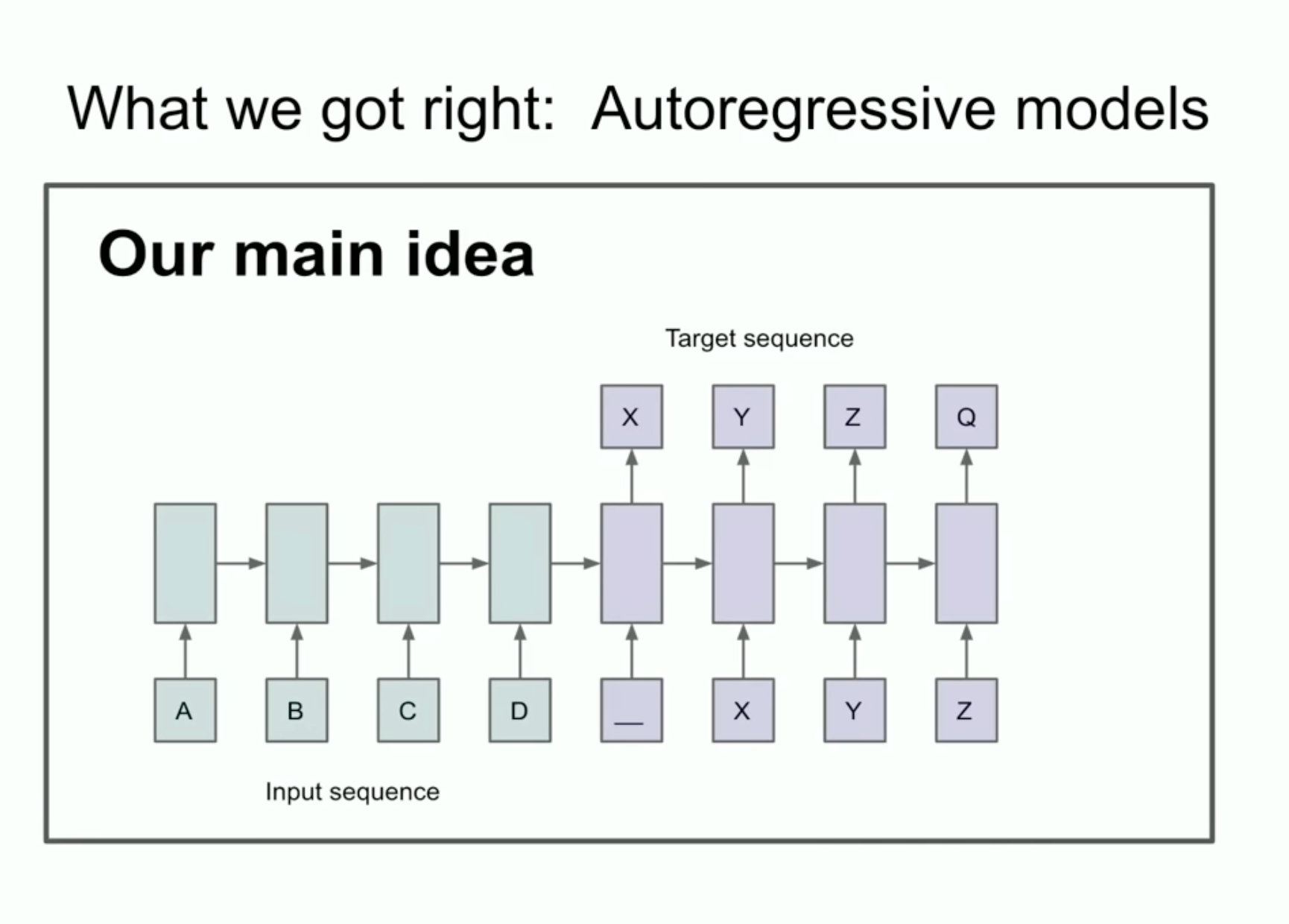
A decade ago, the young AI pioneer also presented an early autoregressive neural network, which was the first built on the belief that "if you train it really well, then you will get whatever you want". The idea is illustrated above.
An autoregressive model takes previous tokens or outputs, such as words in a sentence, as input and predicts the next token as an output.
For example, when fed an input like "the cat sat on the...”, the model predicts the next word will be “mat.”
Sutskever said the most important slide of that earlier presentation sets out an AI scaling law, which states that training big neural networks on massive data sets would inevitably lead to success. Which has turned out to be true - up to a point.
Big data, big opportunities: The brief history of AI scaling laws
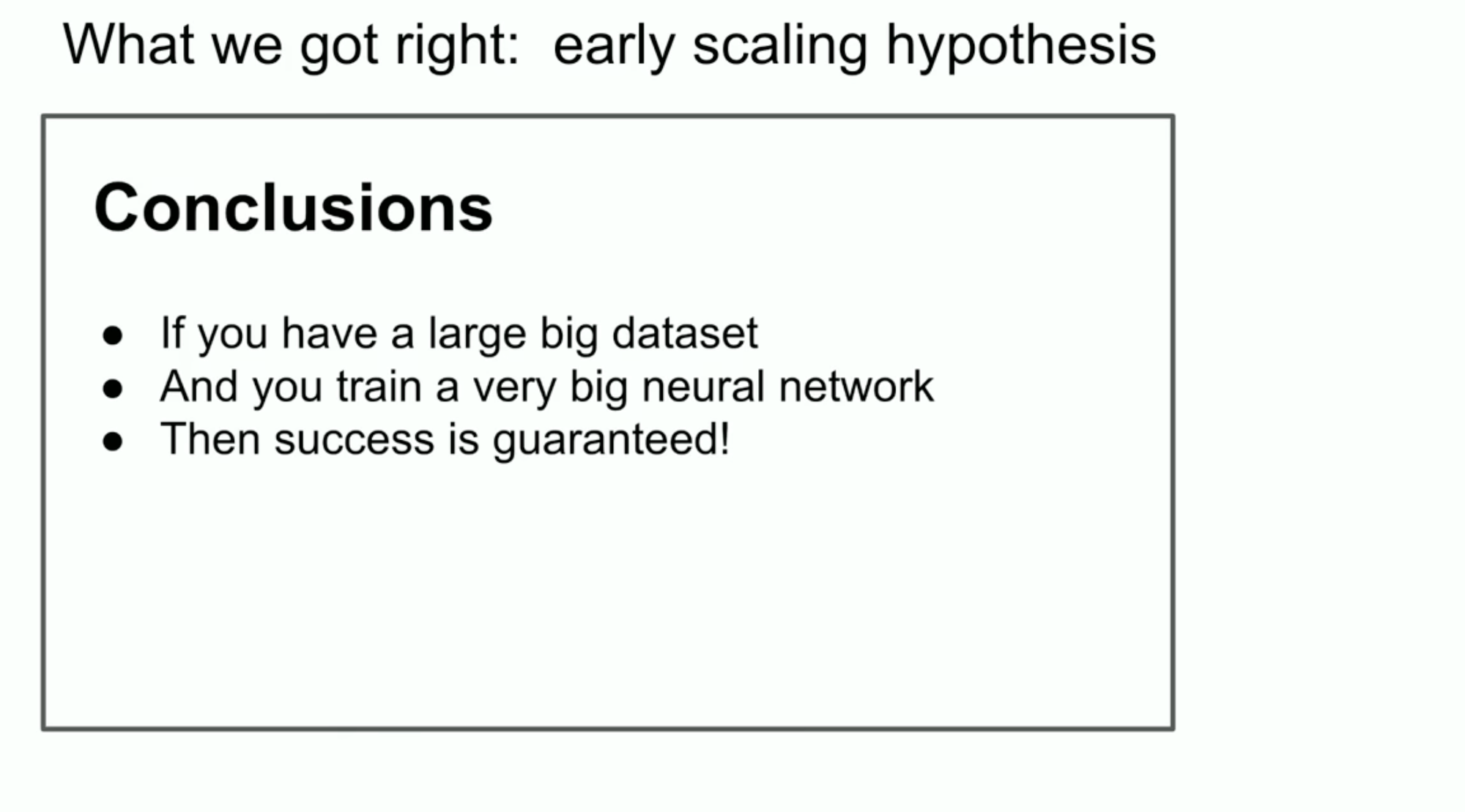
This idea was foundational during the “age of pre-training”, in which large-scale models like ChatGPT munch on massive datasets to produce human-like outputs.
"This has been the driver of all of progress that we see today: extraordinarily large neural networks trained on huge data sets," Sustkever proclaimed.
Pretraining involves teaching a model the foundational knowledge needed to perform its allotted tasks.
However, we're running out of data to feed the machines due largely to the fact that there is only one dataset big enough to train a truly large language model: the internet. And we're not about to get another one of those anytime soon.
The situation mimics that facing the whole of humanity, which depends on a finite resource that will run out one day.
"Pre-training, as we know it, will unquestionably end," Sutskever predicted. "Why? Because while compute is growing through better hardware, better algorithms and larger clusters, the data is not growing because we have but one internet.
"You could even go as far as to say that data is the fossil fuel of AI. It was created somehow, and now we use it, and we've achieved peak data - and there'll be no more [so] we have to deal with the data that we have."
Superintelligence and the far future of AI
Sutskever positioned synthetic data and agentic AI as possible solutions. In the longer term, he predicted the arrival of superintelligence and said the end goal of building an all-powerful model smarter than a human was "obviously where this field is headed.
When we manage to figure out how to make "strangely unreliable" chatbots stop getting confused and hallucinating, we'll move towards systems that are "agentic in real ways" and are capable of reasoning, he said.
However, as models get better, they will also become harder to predict and more chaotic - particularly as they are taught human instincts like intuition or even self-awareness. One sign of this process taking shape is in chess, where even the very best human chess players are finding it difficult to predict the AI's moves.
"The more [AI] reasons, the more unpredictable it becomes," Sutskever warned.
He skated around the risk that such an AI could end up becoming the greatest enemy humanity has ever faced, merely ending on the "uplifing note" that the dawn of AI will create situations which are "very different from what we're used to" and impossible to forecast.
If, of course, we get can around the problems currently facing AI and avoid an AI winter. Get in touch using the link below if you've got any great ideas on how to keep innovation flowing.
Have you got a story to share? Get in touch and let us know.