Big Ideas for 2025: Bridging the AI education gap
"This is a dangerous crossroads. The traditional ways of teaching machine learning through maths are not accessible."
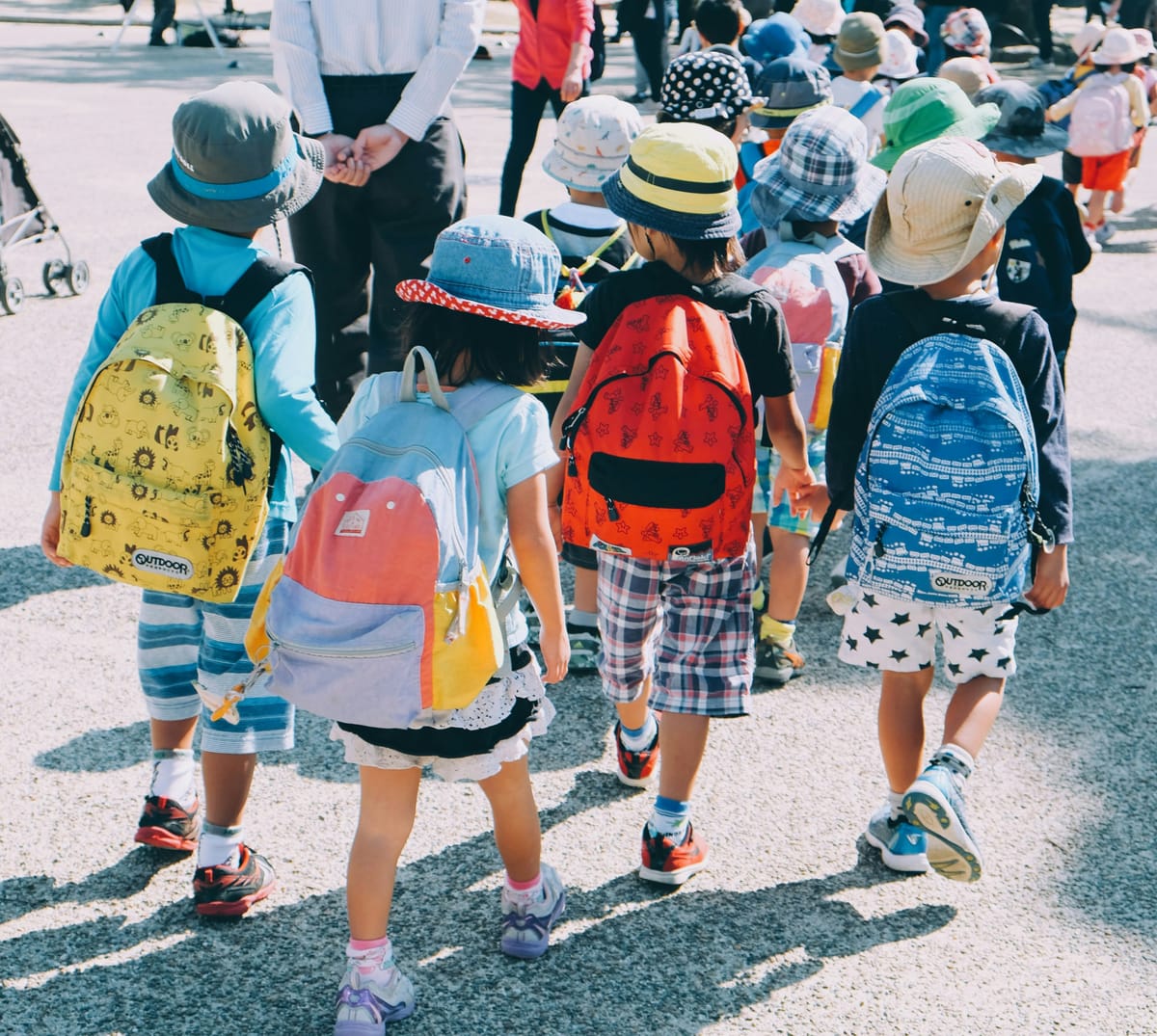
Right now, the AI industry is probably at the same stage of development as the mobile telco sector in the era of the 80s brickphone. But artificial intelligence is not like other product categories.
No matter how good phones were destined to become, there was never any realistic chance that a descendent of the Motorola 8000x handset used by Gordon Gecko would wipe out humanity.
We cannot say the same with AI. There is a significant risk that AI could radically transcend human intelligence and cause p(doom) - an AI safety term for the end of our species.
How do we stop this? Education is at least part of the answer. The more people understand the risks of AI and how it actually works, the better-equipped we are to deal with the threat it poses.
In written comments submitted to Machine, which we've edited lightly, Ash Gawthorp, Chief Academy Officer at the consultancy firm Ten10, warns that the current situation around AI education is suboptimal for businesses, which need to find new ways of teaching staff how to get the best out of AI and protect their organisations against this risks.
"There is a risk of treating AI as an all-knowing entity or magic rather than simply another tool."
"Moving past the magic: Demystifying AI."
Traditionally AI has been taught as an academic subject, starting with the maths underpinning all machine learning (ML) - linear algebra, probability, and calculus - building basic learning models and techniques through mathematic proofs, increasing complexity from the ground up.
Then, over the last couple of years, we saw a huge shift. Instead of this approach, ML and generative AI have been made accessible to the wider population. Like many areas in tech, the deep understanding of how things actually work has simply gone, in the same way it has with the internet or wireless communications.
The difference here (and a dangerous one) is that it's easy to assume that AI is actually intelligent. It's not, or not yet anyway. Without any understanding of how the underlying tech works, people will simply believe this is magic.
The great science fiction author Arthur C. Clarke put this far better than I can: "Any sufficiently advanced technology is indistinguishable from magic."
This is a dangerous crossroads. The traditional ways of teaching ML through maths are not accessible to many people, but nonetheless we need a way of demystifying this and saying: "It's not magic it's just maths."
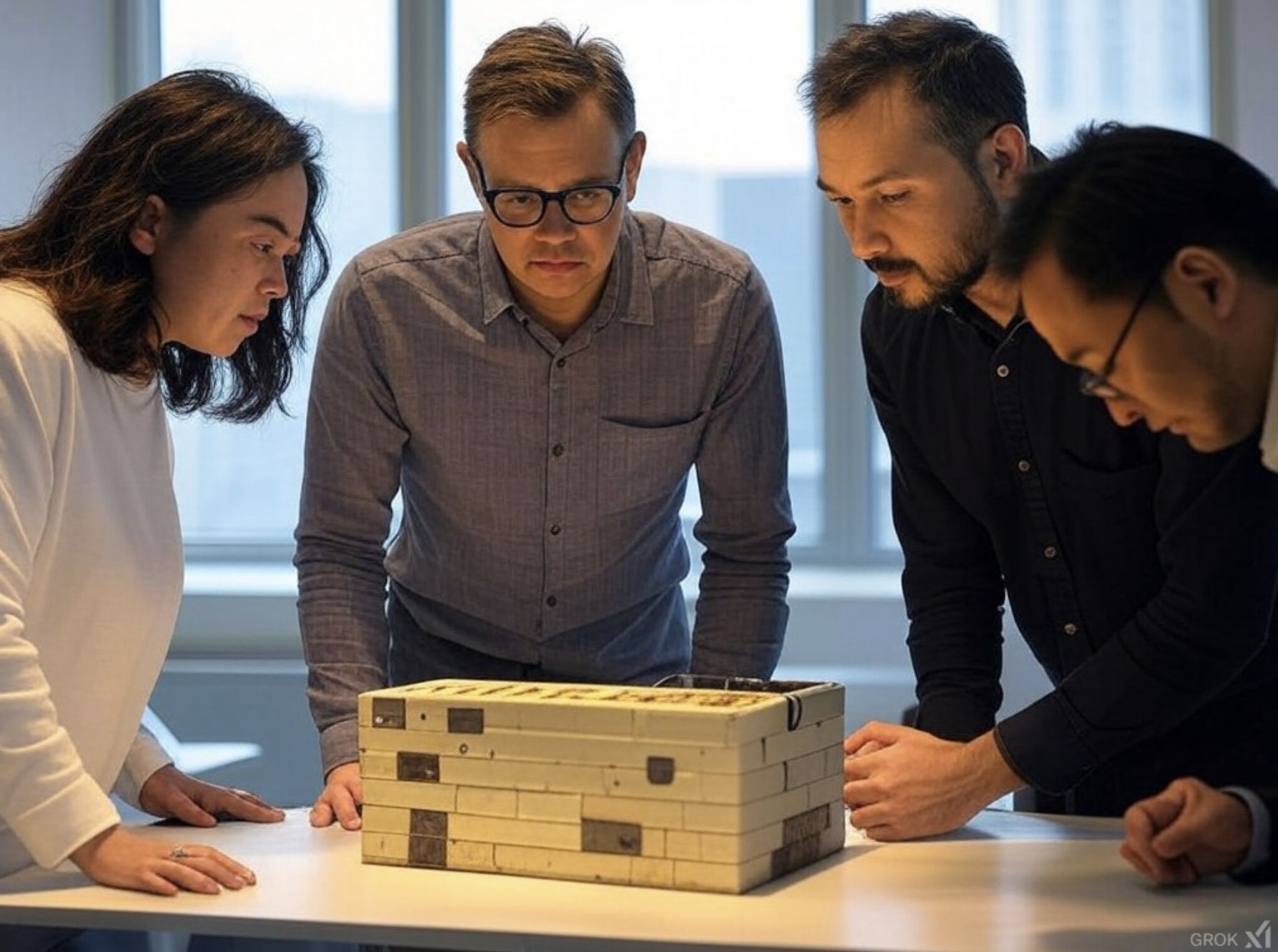
Understanding the machine: New approaches to AI education
Understanding at an intuitive level the basics of ML models, how they are trained, the importance of data, and most importantly understanding their limitations and restrictions is critical. Without this there is a risk of treating AI as an all-knowing entity or magic rather than simply another tool, with its own uses, limitations—requiring governance to ensure it is used safely and appropriately.
I expect to see a pyramid approach to AI knowledge becoming standard practice across organisations. At the base level, every employee will need fundamental understanding of AI's capabilities and limitations - not just what it can do, but critically, what it can't do. This foundation will be essential as AI becomes more pervasive in daily operations.
This base knowledge isn't just theoretical - it's about practical understanding of when and how to apply AI tools appropriately.For instance, knowing when to trust AI outputs versus when to verify against original sources, or understanding the risks of using LLMs with sensitive business information. This foundational knowledge will become as essential as basic digital literacy is today.
Getting the data right
Additionally, this foundation must include understanding the critical role of data quality and preparation. Organisations need evaluation frameworks to assess AI outputs systematically, much like how financial firms are developing standardised methods to verify model accuracy. This practical knowledge extends beyond just using AI tools, to understanding how data quality directly impacts results.
The nature of tech roles themselves will continue to evolve significantly. We're already seeing positions becoming broader in scope - where once you had distinct roles for developers, testers, and business analysts, we're now seeing these boundaries blur. Individuals increasingly need to wear multiple hats, combining technical expertise with business acumen and collaboration skills. This trend will accelerate as AI tools become more integrated into workflows.
The days of pure technical specialisation are fading (there is still a requirement for it more than ever, but the volume of work and roles requiring this level of expertise is reducing). Just as we've seen the complexity of coding reduce with modern development tools, AI will continue this trend. However, this doesn't mean technical roles are becoming less valuable—rather, they're evolving to require a broader skill set that combines technical knowledge with business understanding and people skills.
One point that is a certainty is that training and deploying AI will continue to be very hardware-heavy, requiring lots of compute and lots of fast storage, consuming a lot of power. Unless you have a very niche requirement, chances are you will be leveraging cloud infrastructure and its tools rather than running your own on your own dedicated hardware—mandating cloud expertise.
"As organisations increasingly deploy AI workloads on platforms like AWS,
technical teams need cloud architecture and dev skills as well as robust engineering processes around data engineering. With industry data showing fewer than 10% of enterprises have successfully deployed AI models in production, the focus must be on building reusable foundations rather than isolated solutions."
Don't let AI destroy junior roles
However, one concerning trend that businesses must guard against is the temptation to reduce junior positions in favour of having senior staff use AI tools to increase productivity. While this might seem efficient in the short term, it's fundamentally flawed thinking.
These entry-level positions are where future talent develops the essential experience and insights needed to become tomorrow's leaders. Organisations need to think carefully about how they maintain these crucial career-development pathways. Whilst AI might handle basic technical tasks, junior technical roles nowadays provide crucial experience in managing conflicts, handling customer interactions, and understanding business operations. These experiences form the foundation for future leadership, regardless of technological advances.
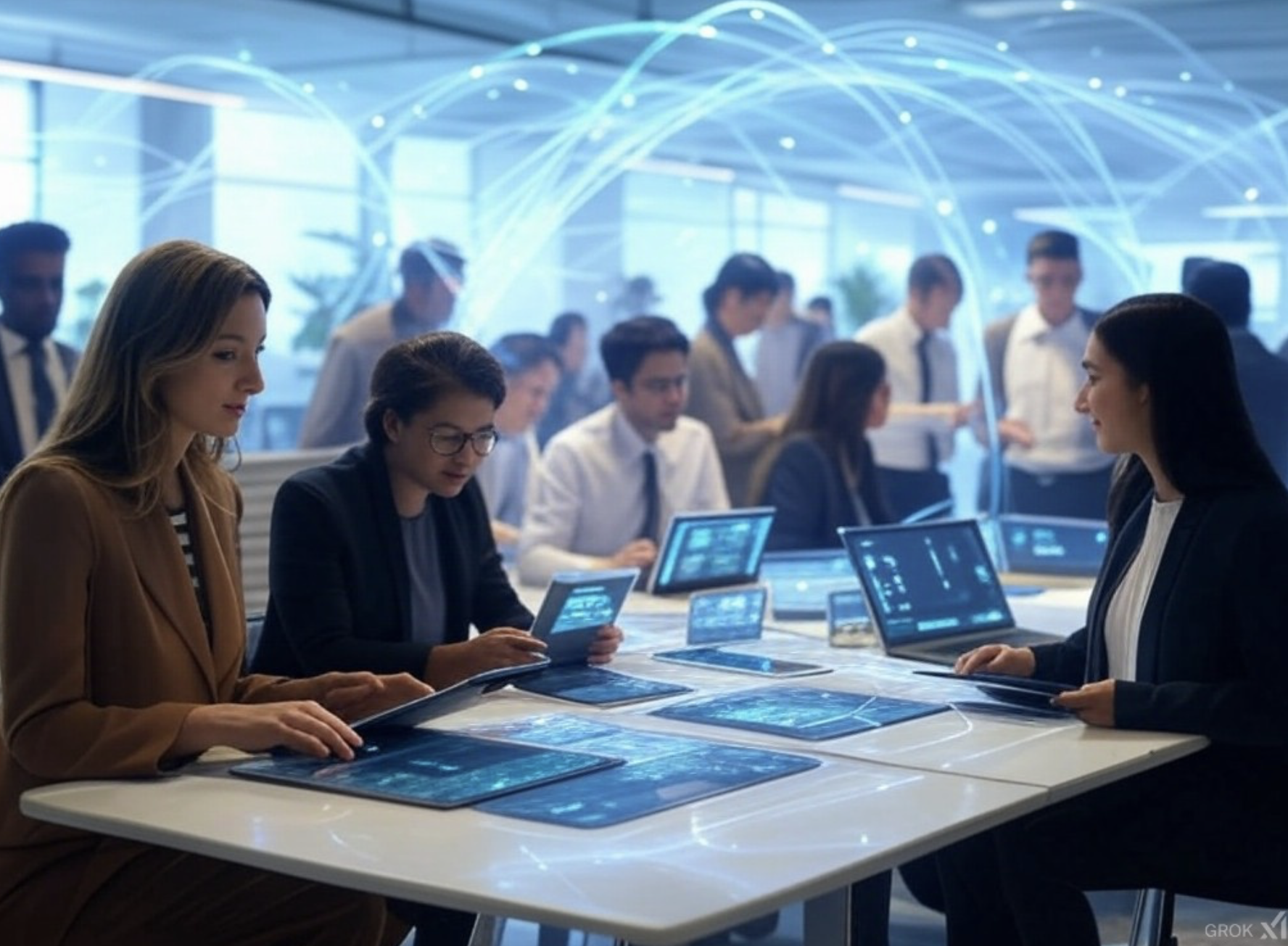
Breaking down departmental silos
Collaboration will become increasingly critical. The traditional separation between 'technical' and 'business-facing' roles will continue to dissolve. We're already seeing this in practice, with meetings now bringing together people who would never have been in the same room ten years ago. This cross-functional collaboration is lauded as the norm - but in reality, it is still often lacking, with mistrust between the 'business' and 'technology'.
True collaboration at this level rather than just talking about it offers a significant efficiency benefit, but it isn't just about efficiency - it's about creating better products and services. When development teams include diverse perspectives, from technical experts to business users, the end result better serves all stakeholders. We've seen this particularly in app development, where inclusive teams create more effective solutions.
This collaboration must now extend to building scalable, platform-based approaches. Businesses need shared technical foundations that can grow with their needs, particularly when managing AI workloads across cloud infrastructure. This includes treating proprietary data as a strategic asset - organisations must develop sophisticated approaches to handling both structured and unstructured information while maintaining security and quality.
We're seeing this particularly in how companies integrate their own institutional knowledge leveraging pre-trained AI systems, creating more effective solutions with a deep understanding of an organisations own processes, data and systems.
Talent in the age of automation
Finally, we'll need to rethink how we assess and develop talent. Rather than looking for specific tool expertise, which can become outdated quickly given the pace of tech evolution, organisations should focus on identifying individuals with the right aptitude and attitude for learning. The key will be finding people who can adapt and grow as these technologies evolve, rather than those who simply tick today's requirement boxes.
Traditional academic pathways, while valuable, shouldn't be the only route into tech careers. We need to recognise that some of the most innovative problem-solvers might come from non-traditional backgrounds. The focus should be on identifying those who can see solutions where others can't, regardless of their formal qualifications.
The organisations that succeed will be those that focus on demystifying AI while building diverse, collaborative teams that bring together different experiences and perspectives through scalable platforms and sustainable practices. It's this combination of technical capability and human insight that will drive genuine innovation and sustainable growth.
Have you got a story or insights to share? Get in touch and let us know.