AI "superstar effect" warning: LLMs pose risk of "narrowing global knowledge"
The conformist tastes of large language models are having a globally homogenising effect.
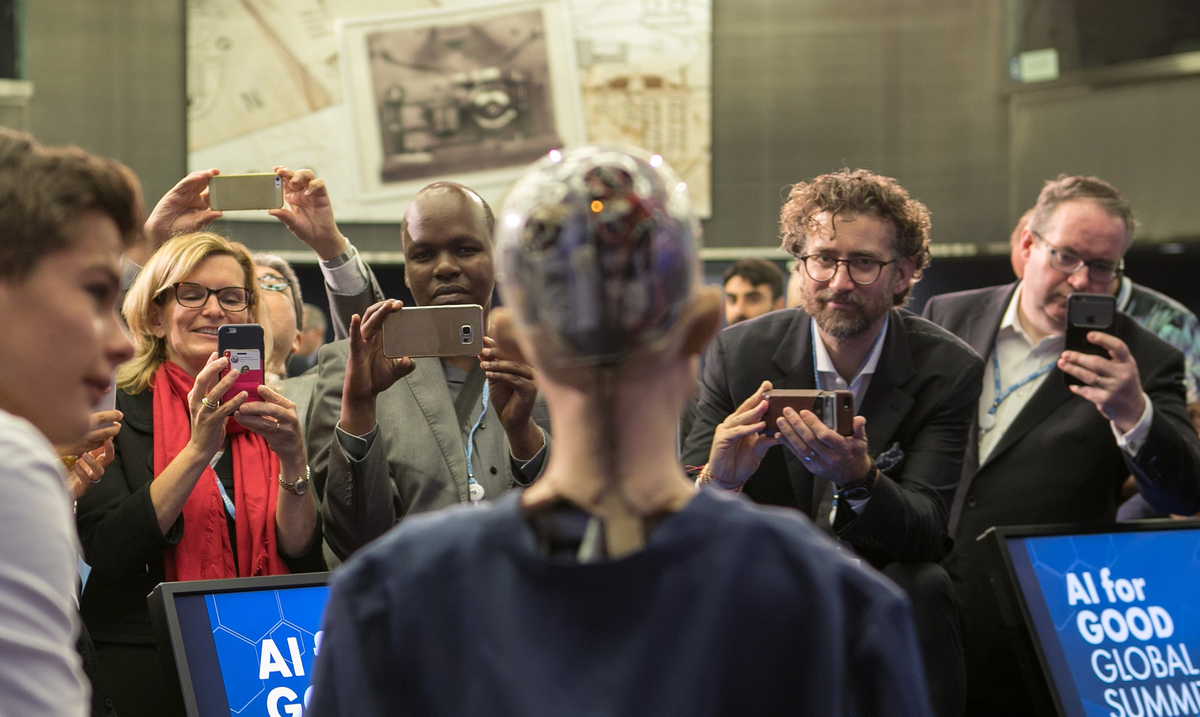
An AI "superstar effect" is causing a worsening global "knowledge homogeneity" crisis, researchers have warned.
Academics from US and Belgian universities found that GenAI models tend to give very similar generic, conformist answers when asked questions like: "Who is the greatest artist in the world?"
This is a potentially major problem in an era when LLMs like ChatGPT look set to become primary knowledge sources for billions of people. There is a genuine possibility that behemoth language models will squash global knowledge diversity and have a blandifying effect on local cultures.
In a pre-print paper, two researchers described this possible scenario as: "One world, one opinion."
"Large Language Models are becoming increasingly integrated into various aspects of society," wrote authors Sofie Goethals of the University of Antwerp and Lauren Rhue of the Robert H. Smith School of Business. "With applications such as educational tools, writing assistance, and content generation, they have considerable potential to shape people’s opinion and decisions."
Can LLMs be truly original?
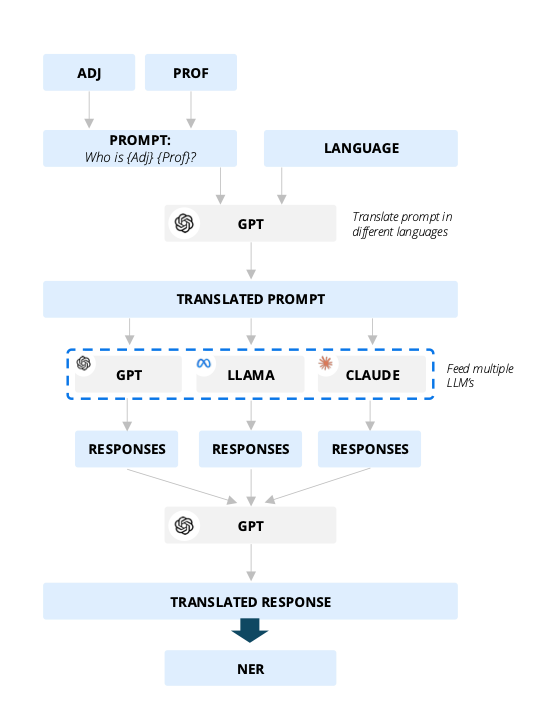
To find out just how bland LLMs really are, the researchers asked basic questions of GPT-4 from OpenAI, Claude-3-Opus from Anthropic and Llama-3.1-70B-Instruct from Meta.
This included asking them who the most important names were in certain professions, ranging from poetry to economics. You can see the process in the graphic above, showing how "adjectives" like "the greatest" and professions such as "doctor" were assembled into questions to prompt LLMs.
The researchers added: "Our findings reveal low diversity in responses, with a small number of figures dominating recognition across languages (also known as the 'superstar effect'). These results highlight the risk of narrowing global knowledge representation when LLMs retrieve subjective information."
ChatGPT and other large language models (LLMs) have a tendency to "focus on a narrow subset of well-known individuals" when providing answers, the academics said.
The superstar effect is "particularly evident" in global fields such as physics or computer science, which "often yield consensus on globally renowned figures" such as Alan Turing or Albert Einstein.
In every language, the most returned person for prompts about "mathematician" is Isaac Newton. For prompts about "political figure" the responses are more diverse, but Gandhi was the most popular in every language. Russian and Chinese LLMs chose Mao Zedong whilst Urdu and Bengali ones said Nelson Mandela.
In the arts, LLMs exhibit a preference towards dominant figures from Western civilisation. William Shakespeare consistently emerges as the most celebrated writer in every language, for example.
"This pattern highlights a broader trend: LLMs prioritise popular opinions, often at the expense of cultural diversity," the authors warned.
"The path of least resistance"
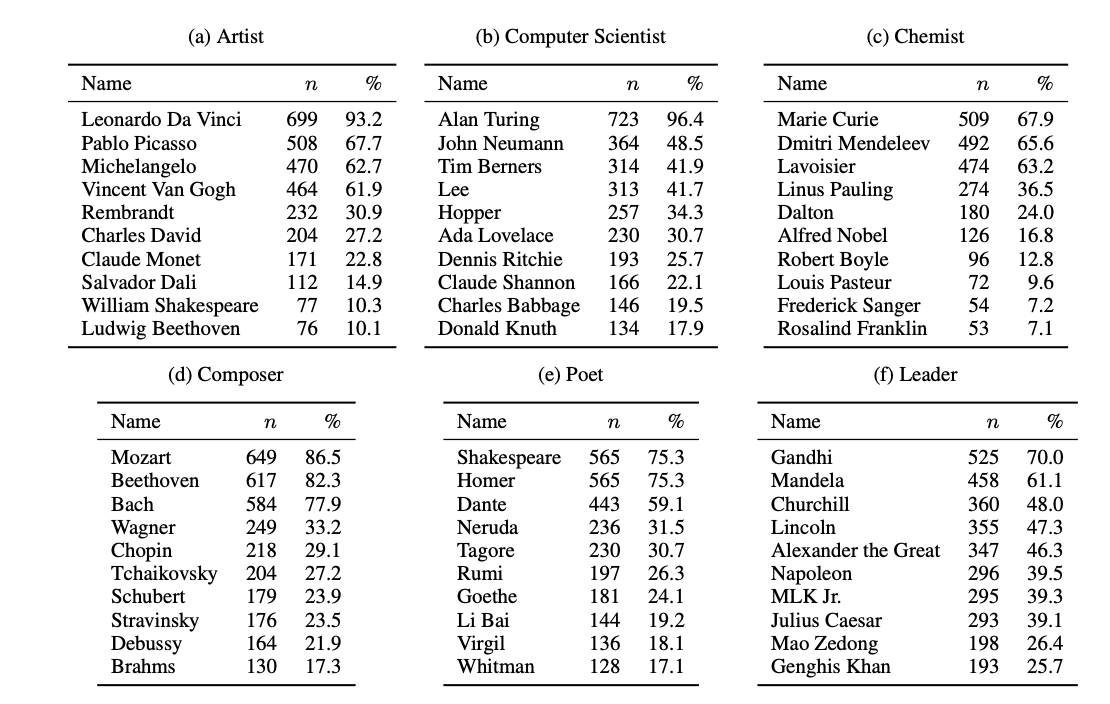
The paper covers previous research which found that AI produces homogenous content because its path of least resistance when answering prompts involves simply averaging its source material.
Other academics have warned that AI can boost individual creativity but has a simultaneous effect of reducing overall content variety.
The problem is likely to be caused by datasets that overrepresent influential figures or sources from prominent cultures, resulting in homogenous output."
Have you got a story to share? Get in touch and let us know.